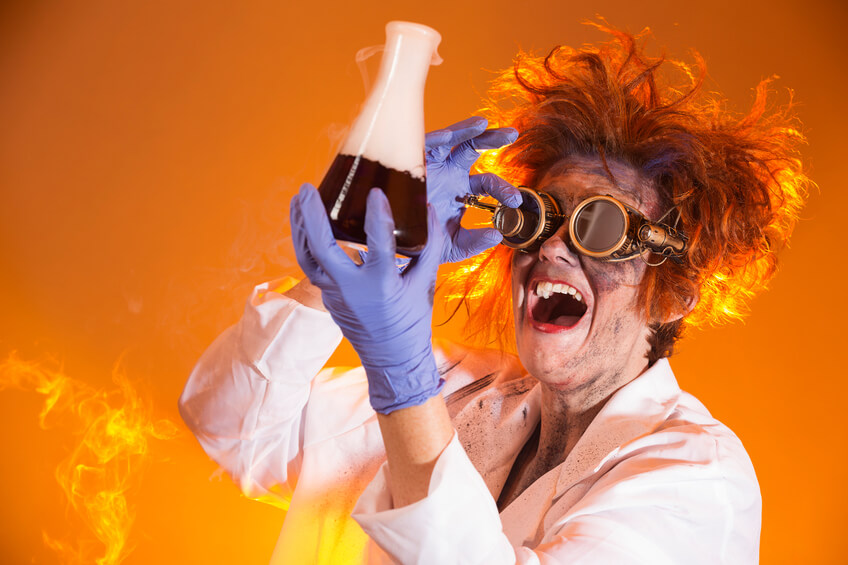
In September, 2016, I will present a webinar about a priori power analysis for determining a proper sample size, a very important aspect in the design stage of a study. We will see how a sample that is too small will result in an inability to see significance that is truly present in our data. That is a waste of both time and money.
But did you know that too large of a sample can also be a problem?
An Example to Consider
Once I performed a simple independent samples t-test for a client who wanted to compare two types of instruction (let’s say Type A and Type B) on the dependent variable of student test scores. The two instructional groups were not large and the total sample size was 46 students. The mean grades were not statistically significant between the two instructional groups of Type A [n = 20; M = 53.05, SD = 25.84] and Type B [n = 26; M = 51.15, SD = 25.38; t (44) = 0.25, p = .804].
For those of you who are novices to statistical lingo, don’t get too involved right now in all of those numbers. Just pay attention to the two mean (average) scores of 53.05 vs. 51.15. That is not a big difference at all. So, the difference shouldn’t be statistically significant. And the p-value was .804, much larger than the p < .05 we’d set at the value to beat. This finding made sense.
Effect Size, Power, and Statistical Significance
Often when one observes non-significant findings, a post hoc power analysis is performed to investigate the power of the test for detecting the effect size of the difference you did find. The effect size, the size of the effect of the mean difference between the two student groups, was d = .07. (Again, don’t worry too much about how we got that effect size, just see that it is teeny). In fact post hoc power for the teeny effect of the mean difference between the test scores of the two groups of students was estimated at only 8%.
Power Defined
Power is the ability to see significance that is truly there.
I like to think of power as a flashlight, and significance is hiding in the corner of a dark room. If my flashlight has 8% power then there is a 92% chance (100% – 8%) that I won’t see the significance. This is called Type II error.
By the way, 50% power means we’d have a 50/50 chance of seeing the significance hiding in the corner. So we need to get a large enough sample to have more than 50% power for our study, or else why bother?
A guideline for acceptable power ion most studies is 80%. At 80% power we have a good chance of seeing the significance hiding there in the corner.
My client asked, “Does it make sense to report my findings, since the sample size was so small?”
I advised her that it wasn’t about the sample being so small. The mean difference between the two student groups was less than two points (53.05 – 51.15 = 1.9). A 2 point difference shouldn’t be significant now should it? And the statistical test indicated this.
My answer was yes, definitely report the findings. Just don’t expect to get them published.
So, we can just get a great big sample and I’ll find significance, right?
Right, unfortunately. So we need to be careful. Let’s look back at our study. In order to detect a significant effect of d = .07 for the mean difference between the teacher groups at 80% power, a total sample size of 5,050 students would have been needed. We had only 46 students. Not nearly enough to detect significance at such a small level of effect. But if we had 6,000 students the p-value would most likely fall beneath the p < .05 level needed to say the mean difference was significant.
However, do we want to really say an effect of 2 points is important? There may be studies where indeed a 2 point difference, or any very small difference, is important! But if you want to test for such small effect sizes, you better plan on collecting a very large sample.
And please don’t ever pull a super large sample just to achieve a low p-value to guarantee statistical significance. It is easier to do in the age of Big Data, but not prudent or ethical to do.
The Problem with Overpowering
A problem with the frequentist statistics we use for hypothesis testing is that the larger your sample, the more you will see significant results. If we had a sample of over 5000 students we would most likely have concluded that a 2 point score difference was statistically significant. But if the effect is that small, we shouldn’t be finding it to be significant.
For our example, an a priori power calculation would have shown that a total sample size of 64 would have given us the ability to see a medium effect size (d = .50) with 80% power at our standard 95% level of significance. So the sample size of 46 was a bit too small, but certainly 5000 students would not be needed. Think about the time and money to collect that much information!
Statistics is pretty awesome when you look at things like this! We don’t want to under or over-power a study; we want it just right to be able to see significance on the effects that matter.
Free Webinar: Back-to-Basics IV: Power Analysis – a priori techniques
Want to learn more? Maybe ask some questions? I’d love to “see” you at our free webinar on September 21, 2016. Sign up here:
https://attendee.gotowebinar.com/register/8311328612805936642