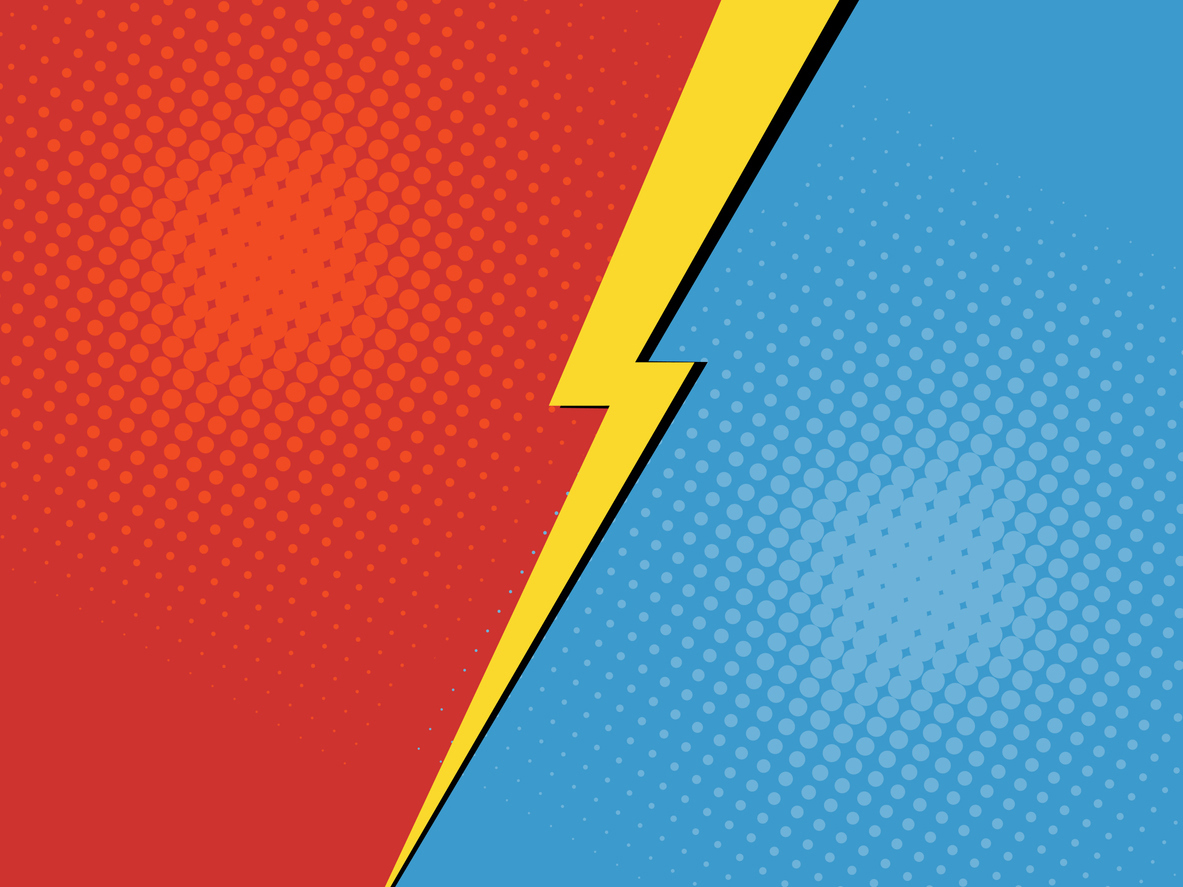
Let’s say you would like to estimate the efficacy of a treatment or intervention but you don’t have the time, money, or other resources to design and implement an actual study. However, there are studies that have been performed by others. These studies can be reviewed and mined for information that will help you to investigate treatment effects. This is where a meta-analysis comes in handy.
In essence, a meta-analysis is an analysis of analyses. You can take the information obtained from a systematic review of the literature and obtain a pooled effect size and associated confidence interval for the treatment of interest.
Recently I worked on a rather large systematic review and meta-analysis of articles for a study on a therapeutic intervention for patients with dementia. The studies included a treatment group (sometimes more than one treatment group), and a control group, as well as baseline measurements, and one or more follow-up measurement times.
The outcomes of interest included continuous level measurements obtained from many different scales and survey instruments. Some scales/instruments were scored so that higher scores indicated improvement in functioning, whiles others were scored so that lower scores indicated improvement.
Deriving a pooled effect of treatment when there are numerous variations on measurement times, outcomes, and directions of the effects can be difficult. I needed to use special techniques to derive effect sizes and standard errors for each individual study and outcome. I ended up using standardized mean differences (SMD) which I could obtain from F-statistics, eta-squared values, or, if I was lucky, and the information was available, from the means and standard deviations for each group (treatment vs. control) at baseline and at the end of each study.
Oh, and many times, a single study would have numerous outcomes. What fun!
You’d think that computing the effect sizes for a conglomeration of studies with various designs, methods, and measurements would be the hard part…it wasn’t too bad once I figured it out. Here are some references and resources I used for computing the effects:
The hard part was finding a way to compile the effect sizes into the pooled effect AND to design pretty forest plots that showed both (a) the individual studies and (b) the individual tests (outcomes) that were nested inside of each study.
Many online resources and effect size calculators involved computing odds ratios from count data. But I had SMD’s from continuous data. Bummer…
There are some very nice meta-analysis functions in Stata but I really struggled with getting the forest-plots to look pretty. I MUST HAVE PRETTY! And preferably without too much coding, gnashing of teeth and hair-pulling.
There is a software, Comprehensive Meta-Analysis (CMA), which offers a free trial. And I tried the trial and it is very comprehensive! But again, I couldn’t get the forest plots to look the way I wanted them to look
Finally, I came across an Excel add-on called MetaEasy, by Evangelos Kontopantelis and David Reeves.
Excel? Really? Oh yes. And it was awesome. And FREE, my favorite four-letter word. The add-on was easy to install, the instructions were easy to follow. And the forest plots were very pretty.
Here is a link which includes the download for MetaEasy, an instruction manual, and an Excel spreadsheet with example data.
Here is a link to an example Excel spreadsheet I made using MetaEasy. In honor of the great Stan Lee I decided to compile it with studies of 11 of Mr. Lee’s superheroes with outcomes of improvement in mental health conditions based on the article, “10 Mental Health Problems Superheroes Suffer” I just made this up for demonstration purposes kiddos. So I don’t wish to discuss the details of who has what or why. Just have fun with it.
I often find that if I keep digging I will hit treasure (not always, sometimes I puncture a sprinkler line). And I am happy to share this lovely golden nugget with you. Enjoy!